Abstract This study aimed to design and test a COVID-19 surveillance system model for community-industry population. A prospective cohort study was conducted from May to December, 2020. Researchers designed a COVID-19 surveillance system and presented it to stakeholders from the community-industry setting in Lamphun and Chiang Mai provinces, Thailand. The model was adjusted following feedback and tested. The model was an Active surveillance for early Alert and rapid Action using Big data and mobile phone application technology for a Community-industry setting (3ABC model). The major components were active surveillance, community-based surveillance, event-based surveillance, and early warning and rapid response. A drive-thru testing unit was operated to enable early detection. Alerts and recommended action on individual and administrative levels were sent via an application and networks. In the testing of the model, risk assessment was initially conducted with regard to COVID-19 transmission in the factories. Researchers provided recommendations based on findings. The improvements included human resource management, systems, and structure. The 3ABC model work well as designed. The participants actively reported events daily including prevention and control activities, animal diseases (foot-and-mouth disease in buffalos and hog cholera), human diseases (dengue and chikungunya), and absent of COVID-19 outbreak. Only five quarantined COVID-19 cases whom were monitored. Daily reports of no abnormal event was also high (70.2% to 71.1%). It is practical and feasible to implement the 3ABC model in a community-industry setting. A further study for a longer period to verify its level of effectiveness should be done.
Keywords: Infectious disease, Epidemic model, Surveillance, Mobile application, Model evaluation
Citation: Thaikruea, L., Srikitjakarn, L., Chakpitak, N., Pornprasert, S., Ouncharoen, R., Khamduang, W., Kaewpinta, B., Pattamakaew, S., Laiya, E., Chanaim, S., and Wongyai, J. 2022. Model of COVID-19 Surveillance System for a Community-industry Setting. CMU J. Nat. Sci. 21(1): e202206.
INTRODUCTION
The World Health Organization (WHO) announced that Coronavirus Disease 2019 (COVID-19), caused by severe acute respiratory syndrome coronavirus 2 (SARS‑CoV‑2), was a pandemic on March 11th , 2020 (World Health Organization, 2021; World Health Organization, 2020a). It has had a huge effect on many countries in the world, including Thailand (Janjua et al., 2021; Marome and Shaw, 2021). Thailand dealt with COVID-19 quite well in the first wave (Gopinath, 2020). A lockdown strategy was effective in the initial situation when there was a lack of COVID-19 knowledge and people were panicking and were afraid of being infected. This strategy may not be effective in the long term and is prone to negative consequences, including the burden of other diseases, mental health problems, economic loss, and social disruption. One important key element of businesses reopening is an active surveillance system with clear specific practical prevention and control responses. The concept is that everyone is eligible to be involved in the surveillance system and contribute to prevention and control responses. It is very detrimental to the economy and difficult in practice to prevent everyone from infection. However, it is possible to prevent further spreading by early warning and rapid response. Fear and stigmatization are of great concern because these factors affect the validity of information in the surveillance system. Furthermore, politically sensitive and mass media attention can make the situation worse. These complex issues were similar to those of the lethal jellyfish health threat situation (Namwat, Suphanchaimat et al, 2020; Thaikruea, 2020). The appropriate surveillance system and prevention and control measures for COVID-19 would be a good solution in fighting complex political sensitive health problems like the lethal box jellyfish stings experience in Thailand (Thaikruea and Siriariyaporn, 2018; Thaikruea, 2020). During the second and third waves of the COVID-19 pandemic, Thailand has been faced with the spreading of the virus from employees working legally and illegally in factories. In addition to the employees, there are families and communities surrounding the industrial estates. This community-industry population is an open cohort with movement between the three units of factory, family, and community. In situations with limited human resources of health personnel and difficult access to the factories, an appropriate surveillance system would be useful for the situation that was complex and dynamic with little knowledge of COVID-19. This study aimed to develop and test a model of a COVID-19 surveillance system for a community-industry setting. The proposed model may be useful for application in other community-industry settings within a similar context.
MATERIALS AND METHODS
A medical epidemiologist from the Faculty of Medicine of Chiang Mai University proposed the concept of the model to the director of the Participatory One Health Disease Detection (PODD) Centre in February, 2020. They invited the Deans of the International College of Digital Innovation, who are responsible for Big data technology, and the Dean of the Faculty of Associated Medical Sciences, who is responsible for the Drive-Thru COVID-19 testing center, to collaborate in the design of a model COVID-19 surveillance system. This team applied for ethical approval and then conducted a cohort study from May to December, 2020. They developed and improved the PODD application (on a mobile phone) to use for COVID-19 surveillance (PODD-COVID-19), including, risk factors for infection (people/places/time) in order to automate data processing for notifying key persons (https://www.opendream.co.th/en/project/podd-en ).
The team proposed the model and discussed the key features with stakeholders, including employees’ leaders, factory owners, community leaders, and authorities from local and provincial governments. The model was adjusted according to their needs and capabilities.
Test the model
Testing sites
The testing sites were forty-four sub-districts that covered two factories in Lamphum and Chiang Mai provinces. There were 187335 households accounting for 348696 people. The first factory approached was located in Chiang Mai province, Lanna Agro Industry Co. Ltd. (LACO). Since 1993, LACO has supplied frozen edamame, vegetables, fruits, and processed-food products to customers around the world. Primarily LACO produces frozen edamame (green soybeans) for export along with many other processed foods. LACO has worked together with local people to support agriculture with a price insurance system. LACO have more than 2000 contracts with farms. The factory has six buildings. The average number of employees was about 3000 per day at the time in question. The second factory was located in Lamphun province, Thai President Foods Plc (TF). TF is the manufacturer and distributor of instant noodle products. TF produces instant noodles under the brand MAMA and products are available in over 60 countries around the world. The factory has four buildings. The average number of employees was about 3500 per day at the time of the study.
Testing steps
The team conducted a risk assessment in regard to potential transmission and outbreak of COVID-19 in factories and provided recommendations for improvement based on their individual contexts. Daily reports of no abnormal event and illnesses relevant to other endemic diseases were included in order to test the model. The illnesses were defined by signs and symptoms into four categories, specifically fever with respiratory symptoms and signs, fever with skin lesions, gastrointestinal symptoms and signs, and other symptoms and signs. Data collection was done using the PODD-COVID-19 application.
Ethics
The study (Study code: COM-2563-07241) was approved by the Research Ethics Committee No.4, Faculty of Medicine, Chiang Mai University (No. 182/2020).
RESULTS
Designing the model
The proposed model of the COVID-19 surveillance system had a combination of Active surveillance for early Alert and rapid Action using Big data and mobile phone application technology for Community-industry setting (Short name ‘3ABC’). The major components of this system were active surveillance, community-based surveillance, event-based surveillance, early detection and alerts using technology tools, and automatic feedback for initial rapid action. The feedback for initial action was based on the capabilities and resources in the communities. Further action, if needed, was supported by the higher level of government that was responsible for the community-industry population. The flow of the 3ABC surveillance system is shown in Figure 1.
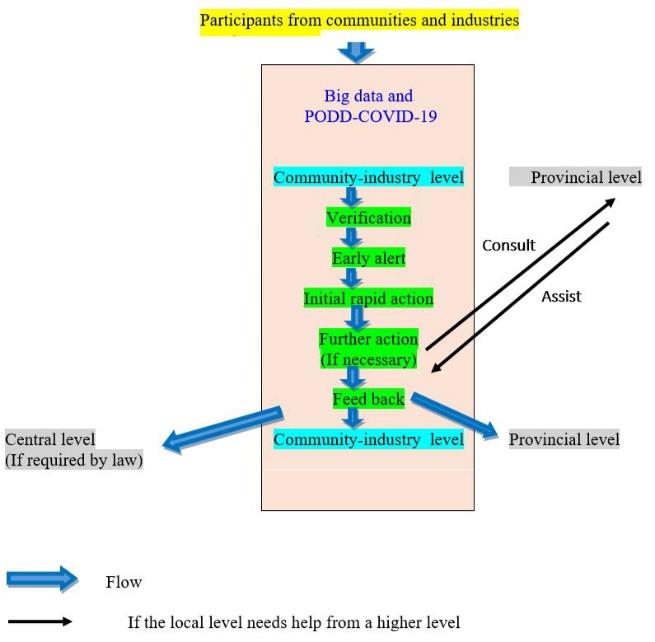
Figure 1. The flow of the 3ABC surveillance system.
Source of data
The source of data was from community-industry population using the PODD-COVID-19 application. The team invited all stakeholders in the tested area to participate in the study, including health personnel from district health centers and provincial health offices, employee leaders, factory owners, human resource managers, community leaders, and village health volunteers. Village health volunteers were villagers who volunteer for public health tasks in the villages. The community-industry population who participated in the study (Participants) were divided into two groups defined by roles, specifically administrators and reporters. Reporters in each surveillance network (Lamphun and Chiang Mai Provinces) monitored and reported health related events and illnesses occurring during the tested period using the PODD-COVID-19 application on mobile phones. The data were sent to the data center where the inputs were analyzed and interpreted. When the results triggered the alert criteria, an alert message and recommendations for initial action were sent immediately to the key administrators and reporters who were responsible for initial action (Figure 1).
Laboratory testing
In order to increase early detection, which led to early alert and rapid action, a Drive-Thru COVID-19 testing center was launched. People who wanted to have COVID-19 tests but did not fit the government criteria (due to limited tests) could use this facility. The steps of the Drive-Thru COVID-19 testing procedure included the following:
1. Registration: People at risk reserved a queue position for COVID-19 testing via online application which was available on their mobile phone (https://www.covidtestcnx.org). The clients provided their information and completed a brief questionnaire on the online application. The system generated a client identification code and appointment date, time, and place for sample collection. Clients who met the criteria for free-of-charge diagnosis covered by the Thai government were referred to the government sector.
2. Specimen collection: The Specimen Collection Center was located in the Chiang Mai University Education Center at Lamphun province. Clients visited the center at the appointed date and time. The entire service was provided to the clients without leaving their cars. Clients who came on a motorcycle or walk-in were invited to the specimen collection booth that was controlled with a positive airflow to prevent virus transmission from clients to health care personnel. Also, basic hygiene practices including cleaning of the booth surfaces and changing gloves between each client were performed to avoid client-to-client contamination. Nasopharyngeal and throat swabs were performed strictly within biosafety standard precautions by well-trained health care personnel. This step took approximately 10-15 minutes.
3. COVID-19 testing: COVID-19 diagnosis was reliant on the molecular detection of RNA of SARS CoV-2 virus in diagnosed specimens using a real-time reverse transcription polymerase chain reaction (real-time RT-PCR) technique. The commercial test kit was targeted at the ORF1ab and N genes together with a human endogenous gene to serve as internal controls (DAAN Gene 2019-nCoV RT-PCR Kit). If the first-round result was detectable, the sample was checked again for confirmation with the in-house COVID-19 test kit targeting RNA-dependent RNA polymerase (RdRp) and N genes. This was the protocol available from the Department of Medical Sciences of Thailand and the World Health Organization (World Health Organization 2020b).
4. Result reporting and instructions: The result was reported directly to the client through his/her online application within 6 - 24 hours of specimen collection. Also, instructions with regard to daily practices after COVID-19 result awareness were provided regardless of whether the results were positive or negative. Confirmed COVID-19 cases were notified by phone, had a consultation and were instructed to report immediately to the Department of Disease Control of Thailand for quarantine (required by law). The case was then investigated to check the possibility of transmission to people with whom they had been in contact.
Technology tools
The technology tools that were used for real time tracking and evidence-based management were Big data and PODD-COVID-9. The team identified keywords for detecting COVID-19 from available data sources including social media data (most commonly the LINE and PODD apps), news, and announcements from the authorities. Data via PODD-COVID-19 surveillance, including risk history and reported symptoms, were linked to open data of Big data. When the findings reached the sensor criteria, the system sent alerts and advice messages to each individual via their mobile phone for rapid action. The results of the situation and advice messages were shown on a dashboard for decision making by the administrators (i.e. production managers, plant personnel managers, factory owners, local governments, local and provincial public health officers). The academic team adjusted alerts and advice messages based on current knowledge and regulations/laws. In the case of Big data, analyses included segmentation and mapping of COVID-19 Disease. A segmentation algorithm was used to find words found in the data. Visual analysis of these clusters indicated the prominence of disease terms. For mapping COVID-19 cases, a schematic application of the cartographic process was applied. The objective was to make a continuous map of the entire geographical range of the disease from a sample of locations where the disease was observed. The process started with records of disease occurrence obtained from the reports (Berthe, Bouley et al., 2018; G20 Health Report, 2018).
Testing the model
Risk assessment and improvement
The team trained the participants in use of the 3ABC model. Medical epidemiologists walked through factories to assess the risk according to COVID-19 spread. The team presented findings and advised the owners and managers about improvements. For locker room and uniform room, there were crowded utilization and employees did not wearing masks all the time. The improvements of both rooms included always wearing mask, keep distancing, shift management, one-meter line up in front of the room, and re-arranged clothes rack. For Canteen, the improvement included increasing air flow (fan and window opening), installing alcohol sanitizer station, installing transparent partitions on tables, cleaning schedule, and shift management for lunch time (Figure 2 and Figure 3). For first aid room, the improvement included analyzing data for signs and symptoms of COVID-19, monitoring, and follow-up. The team advised the factories to develop business plan that included preparedness and response to COVID-19 outbreak.
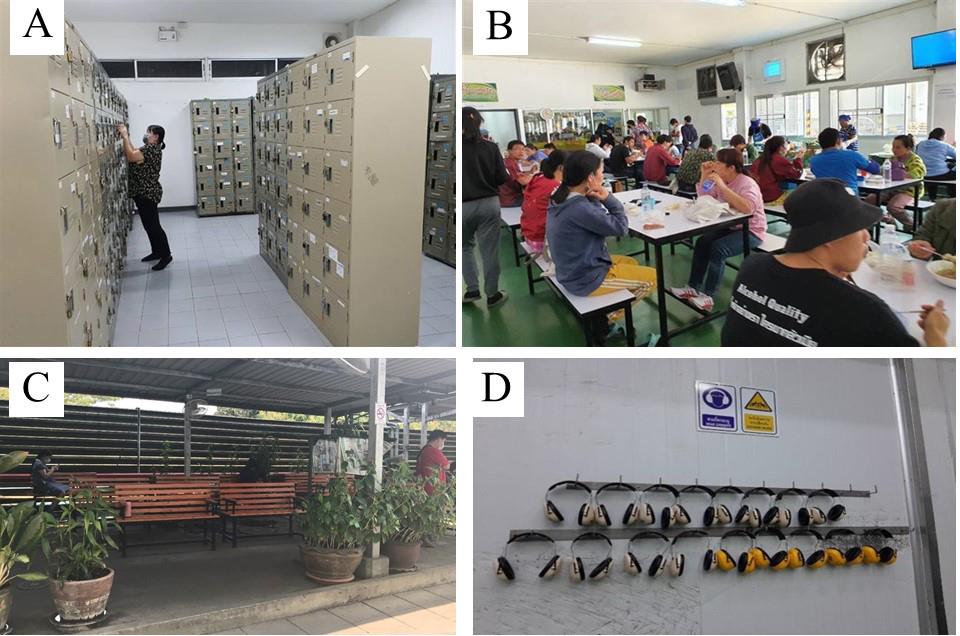
Figure 2. The academic team assessing the factory for risk of COVID-19 spread: (A) Locker room (B) Canteen (C) Resting area (D) Equipment in production line
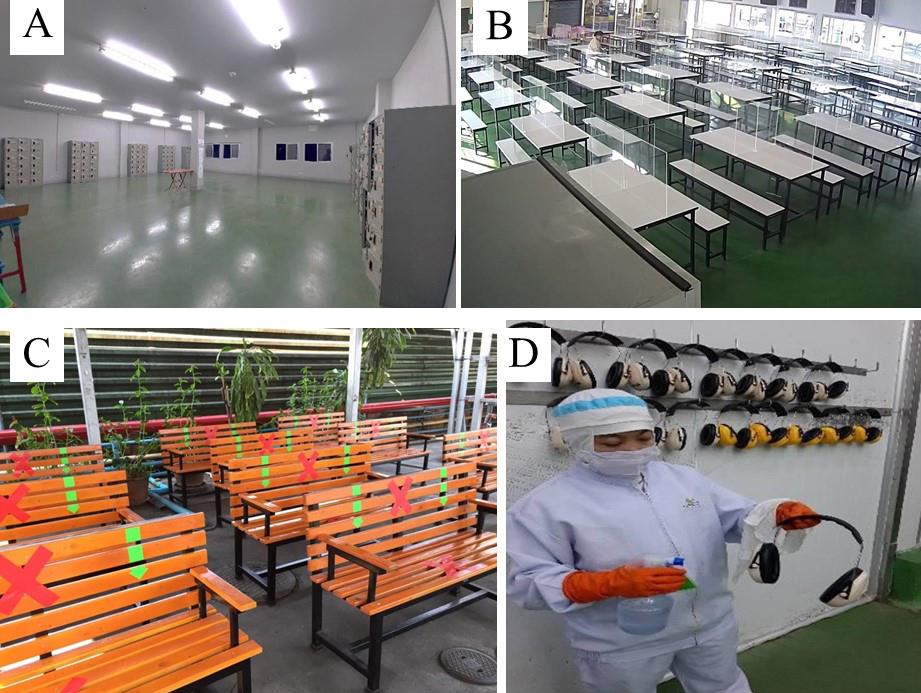
Figure 3. Improvements after recommendations: A) Locker room (B) Canteen (C) Resting area (D) Equipment in production line.
Health related events and illnesses
From July to September, 2010, the participants reported locations and health related activities daily, including detection of human and animal diseases, monitoring of self-quarantined COVID-19 cases, provision of health education, screening villagers who had carried out activities in communities (such as funerals, training, religion-related ceremonies, etc), conduction of interventions (such as eliminating mosquito larvae, spraying mosquitos, environmental surveying, educating about COVID-19, cleaning public places, etc). Reported animal diseases were foot-and-mouth disease in buffalos and cholera in hog. Reported human diseases included dengue and chikungunya. No COVID-19 outbreak occurred in the testing sites. The provincial health officer notified the local health personnel and officers five known cases of COVID-19 whom had to be quarantined in the communities. Participants monitored these cases for 14-day period. Participants reported a high risk contact person who came to stay in the village. Daily reports of no abnormal event ranged from 70.2% to 71.1% (Table 1).
Table 1. The number of isolates obtained from Cipayung dan Cipeucang landfills.
Time and events
|
8-31 Jul 2020
|
Aug 2020
|
Sep 2020
|
|
Human Disease
|
Covid-19
|
Animal Disease
|
No abnormal event report
|
Human Disease
|
Covid-19
|
Animal Disease
|
No abnormal event report
|
Human Disease
|
Covid-19
|
Animal Disease
|
No abnormal event report
|
|
Number of report
|
74
|
3
|
0
|
700
|
189
|
2
|
1
|
851
|
80
|
0
|
2
|
891
|
Number of case
|
94
|
3
|
0
|
|
215
|
2
|
1 Feline
|
|
90
|
0
|
8 Bovine
|
|
80 Swine
|
Incidence per 100,000 a
|
27.0
|
0
|
-
|
|
61.7
|
-
|
-
|
|
25.8
|
0
|
-
|
|
Expected number daily report of no abnormal event b
|
|
|
|
984
|
|
|
|
1,213
|
|
|
|
1,255
|
Percentage of daily report of no abnormal event report c
|
|
|
|
71.1
|
|
|
|
70.2
|
|
|
|
71.0
|
|
Note: b (Number of incident cases of disease occurring in each month/ 348696 population number in 44 sub-districts)* 100,000; Known cases of COVID-19 whom had to be quarantined in the communities. They were not incident cases occurring in the communities.
c Expected number of daily report of no abnormal in each month from 44 sub-districts
d (Number of daily report of no abnormal in each month / expected number of daily report of no abnormal in each month) x100
Illness reports
The report rates of fever with respiratory symptoms and signs, fever with skin lesions and gastrointestinal symptoms and signs were 94.2%, 37.8% and 12.0%, respectively (Table 2). For other symptoms and signs category, the report rate was 75.2% and the top three most reported symptoms and signs were muscle pain (15.0%), muscle pain and headache (11.5%), and headache (8.3%).
Table 2. Number of reported illnesses by the participants from testing sites during the testing period.
Reported illnesses
|
Frequency
|
Percentage %
|
Fever with respiratory symptoms and signs
|
|
|
No symptom and sign
|
23
|
5.8
|
Fever
|
345
|
86.5
|
Fever with sore throat
|
8
|
2.0
|
Fever with cough
|
9
|
2.3
|
Fever with difficult breathing
|
6
|
1.5
|
Fever with cough and sore throat
|
3
|
0.7
|
Fever with chills
|
2
|
0.5
|
Fever, cough, sore throat and sputum
|
1
|
0.3
|
Fever with sputum
|
1
|
0.2
|
Dyspnea
|
1
|
0.2
|
Total
|
399
|
100.00
|
Fever with skin lesions
|
|
|
No symptom and sign
|
248
|
62.2
|
Pethichea
|
95
|
23.8
|
Erythematous wheals
|
41
|
10.3
|
Erythematous rash
|
4
|
1.0
|
Erythematous wheals and pethichea
|
4
|
1.0
|
Erythematous rash and pethichea
|
3
|
0.8
|
Papule
|
3
|
0.8
|
Erythematous rash and erythematous wheals
|
1
|
0.2
|
Total
|
399
|
100
|
Gastrointestinal symptoms and signs
|
|
|
No symptom and sign
|
351
|
88.0
|
Nausea and vomiting
|
35
|
8.8
|
Diarrhea, nausea, vomiting, and stomach ache
|
5
|
1.3
|
Diarrhea and stomach ache
|
3
|
0.7
|
Diarrhea, nausea and vomiting
|
2
|
0.5
|
Diarrhea
|
1
|
0.3
|
Stomach ache
|
1
|
0.3
|
Nausea, vomiting, and stomach ache
|
1
|
0.2
|
Total
|
399
|
100
|
Word clusters
Visual analyses of word clusters were used to indicate the prominence of disease terms. The bigger the size of the words, the higher the frequency of these words were. The words reported the most frequently included fever, fever with pethichea, fever with erythematous rash, and nausea and vomiting (Figure 4).
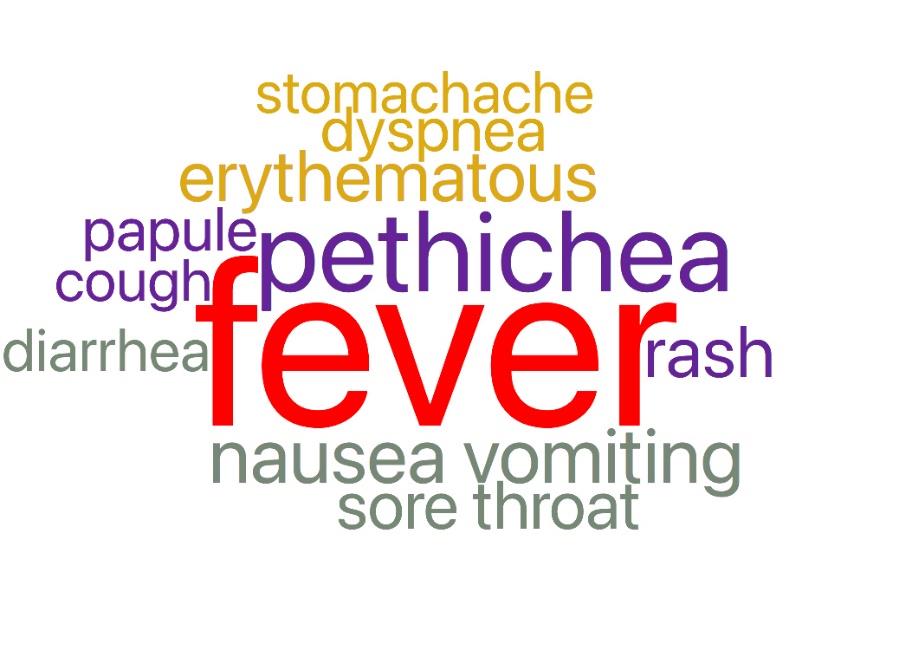
Figure 4. Visual analysis of word clusters indicated the prominence of signs and symptoms.
DISCUSSION
A passive surveillance system gives a high level of specificity when there is sufficient knowledge of that particular disease. However, it does not work well with emerging diseases that spread rapidly. An active surveillance system is good for coverage but more expensive and requires a higher task forces compared to those of passive. Event-based surveillance increases sensitivity but it is prone to false positives. In a rapid dynamic situation with little known about the disease, an appropriate surveillance system is needed. It is also difficult to set up surveillance and prevention and control systems in a unique community-industry setting where the population is an open cohort with regular movement between the three units of factory, family, and community. This proposed model of the COVID-19 surveillance system might be appropriate. This 3ABC model involved the integration of active surveillance, community-based surveillance, event-based surveillance, prevention and control. An early detection process started from incoming reports of abnormal health related events or illness of suspected cases from within the communities. This was done by real time tracking using the PODD-COVID-19 application. An alert message was also automatically sent to the administrators for initial rapid action. The feedback for initial action was based on capabilities and resources in the communities. To reduce cost and increase sustainability, community-based surveillance was appropriate since it was an active process of community participation in detecting, reporting, responding, and monitoring any COVID-19 related health events in the community-industry setting. When COVID-19 clusters become large, it is critical that testing of suspected cases or people who have been in contact, whether symptomatic or asymptomatic, continues so that cases can be isolated, contacts can be quarantined, and chains of transmission can be broken (World Health Organization, 2020c). Early identification of new cases of COVID-19 patients is a strategy recommended by WHO to efficiently prevent the spread of the epidemic and also prevent disease progression to severe disease in active infected patients (World Health Organization, 2020d). This strategy can be achieved by a proactive service system for diagnosis of COVID-19 infection which may be helpful for people who would like to, or need to, know their COVID-19 status and can then have access to the diagnosis. In the beginning of the COVID-19 epidemic when the team conducted this study, it was the recommended practice to use real-time RT-PCR to identify SARS-CoV-2 through detection of its E gene, RdRp gene, and N gene (Yu, Du, et al., 2020). Drive-Thru COVID-19 Testing was one of strategies which had been proved to have a high efficacy in management of massive community outbreaks, such as the one in South Korea (Kwon, Ko et al., 2020). Kwon KT et al. demonstrated that the entire service takes about ten minutes for one client without leaving his or her car. Fortunately, there was no COVID-19 outbreak in the testing sites during the study period. Only five quarantined COVID-19 cases were identified and monitored by the participants in the communities.
This study used the PODD-COVID-19 application, which was modified from the PODD application. PODD is a community-participation surveillance system focusing on getting real time information about people, animals, and places at risk of disease infection, and providing initial management on the risk level of that place. Throughout the past five years, the digital tool of PODD has proven its ability to strengthen the community surveillance system. This technology helps prevent disease transmission and natural disasters by minimizing risks through early detection and rapid response to the abnormal events, such as Avian influenza management and rabies prevention (Yano, Phornwisetsirikun, et al., 2018; Yano, Trakarnsirinonb, et al., 2016). The characteristics of PODD included being a community-owned one health surveillance system. Locals own and can use data directly and detect outbreak signals promptly, data is processed in an automated system and real time notifications are sent to relevant participants at local, district, and provincial level. These features facilitated local governments or communities to respond and perform their complex work. The efficacy of these features of PODD were recognized and it received awards from the Department of Disease Control, the Ministry of Digital Economy and Society, and the ASEAN ICT AWARD 2018. The latest award was the Grand Prize of Trinity Challenge 2021 (£1.3 million) (https://www.trin.cam.ac.uk/news/the-trinity-challenge-winners-announced/Key). The PODD system was mentioned in the report by the World Bank Group, and the G20 Heads of Government and Ministers Meeting in Japan 2019 (Berthe, Bouley et al., 2018; G20 Health Report, 2018). This study modified PODD application to PODD COVID-19 application in mobile phone. The PODD COVID-19 application was practical for the participants with a health professional or smart phone technology background, however, it took time for people who did not have skills in smart phone technology to access the application. In addition, only an android version was available. In this study not all employees in the factories needed to install this application on their smart phones, only the participants whose roles were reporters responsible for the group of employees or households in the testing sites needed to do so. The existing disease surveillance system in Thailand allows only physician to give diagnosis. Health personnel working in community health centers can report suspected case or clinical manifestation. Thus, recognized signs and symptoms of COVID-19 were appropriate for the participants in this study to identify and report. In order to test whether the 3ABC model works, other endemic diseases were included. Similar to those of COVID-19, endemic diseases were categorized into illness groups using signs and symptoms. The 3ABC also included ‘other category’ in case that there would be other diseases occurring during the testing period. The findings supported that the participants actively reported health related events, such as detecting suspected cases of dengue and chikungunya, monitoring dengue and chikungunya outbreaks, implementing interventions, monitoring self- quarantine COVID-19 cases, educating health related diseases, and screening of COVID-19 cases in the communities. Daily report of no abnormal event was necessary for checking whether the surveillance system was active. In this model, the daily report of no abnormal event were high. The research team could not construct a continuous map of the entire geographical range of COVID-19 cases because there was no outbreak during the testing period. Only five quarantined COVID-19 cases were monitored in the communities. The reported symptoms and signs of illnesses and word cluster results were relevant to dengue fever and chikungunya infections. A possible explanation of the feasibility of the 3ABC model included the model used the same structure and available resources in the community, communication in surveillance networks were active, illness written in the mobile application was in layman’s terms, and the majority of participants had experience as village health volunteers.
There were some limitations in this study. This model needed to be used with caution as there needed to be a balance between the need for information and public safety and the right to privacy. However, this study was conducted within the overarching regime of the Communicable Disease Act BE 2558 if it came into force to help dealing with the pandemic in Thailand (https://ddc.moph.go.th/law.php?law=1). This act required individuals and factories to report suspected symptoms of COVID-19, which had been recently included in the list of dangerous communicable diseases, to the authority. The Act also offered authoritative power to ‘Communicable Disease Control Officers’ to implement necessary measures to tackle the outbreak, including inflicting quarantine measures on people at risk (such as the returnees from high-risk countries, or high-risk close contact of confirmed cases) or ensuring isolation of the patients in institution-based care facilities. This study aimed to design a model and test it over a short period, therefore it might not give a guarantee of a long-term outcome. It is worth noting that after finishing the testing model, the researchers have been provided advices for the factories throughout the third wave of COVID-19 pandemic. The strength of this 3ABC model is that it combines prevention (All aspects of structure, system, and manpower), surveillance, and business plan (with preparedness and response of COVID-19). This strength may contribute to long-term outcome. By mid-July 2021, other community-industry settings in Thailand have outbreaks but not in the testing sites used in this study (Siamrath Online news, 2021).
CONCLUSION
The major components of the 3ABC surveillance system for community-industry settings were active surveillance, community-based surveillance, event-based surveillance, early warning, rapid response, prevention and control. The technology tools included Big data and PODD- COVID-19. It is practicable and feasible to implement the 3ABC model in a community-industry setting. A further study for a longer period to verify its level of effectiveness should be done.
ACKNOWLEDGEMENTS
We would like to thank the participants from communities, Lanna Agro Industry Co. Ltd. and Thai President Foods Plc for contributing information and for their cooperation. We are grateful to professional English editor from Research Administration Section of Faculty of Medicine, Chiang Mai University for refinement the English Language.
AUTHOR CONTRIBUTIONS
Lakkana Thaikruea developed concept, designed, conducted the study, analyzed data, and wrote the manuscript. Lertrak Srikitjakarn designed, conducted the study, and analyzed data. Nopasit Chakpitak designed and conducted the study. Rujira Ouncharoen, Woottichai Khamduang, Boontuan Kaewpinta, and Jiraporn Wongyai conducted the study. Sakulrat Pattamakaew, Ekkachai Laiya and Somsak Chanaim conducted the study and analyzed data. All authors have read and approved of the final manuscript.
CONFLICT OF INTEREST
The authors declare that they hold no competing interests.
REFERENCES
Berthe, F.C.J., Bouley, T., Karesh, W.B., Gall, F.G.L., Machalaba, C.C., Plante, C.A., et al. 2018. Operational framework for strengthening human, animal and environmental public health systems at their interface (English). Periodical Operational framework for strengthening human, animal and environmental public health systems at their interface (English), Accessed 21 April 2021, from http://documents.worldbank.org/curated/en/703711517234402168/Operational-framework-for-strengthening-human-animal-and-environmental-public-health-systems-at-their-interface.
G20 Health Report. 2018. A mobile application for early detection of infections at the human/animal interface. G20 Health and development Partnership. 54-57.
Gopinath, G. 2020. The Great Lockdown: Worst Economic Downturn Since the Great Depression. Accessed 7 May 2021, from https://blogs.imf.org/2020/04/14/the-great-lockdown-worst-economic-downturn-since-the-great-depression
Janjua, L.R., Muhammad F, Rehman A., and Yu, Z. 2021. Impact of COVID-19 pandemic on thailand economy and tourist industry: an empirical forecasting using ARIMA. Brazilian Journal of Operations & Production Management 18, 1-3, Accessed 20 Jul 2021, from https://bjopm.emnuvens.com.br/bjopm/article/view/999.
Kwon, K.T., Ko, J.H., Shin, H., Sung, M., and Kim, J.Y..2020. Drive-through screening center for COVID-19: a safe and efficient screening system against massive community outbreak. Journal Korean Medical Science. 35: e123.
Marome, W. and Shaw, R. 2021. COVID-19 Response in Thailand and its implications on future preparedness. International Journal of Environmental Research and Public Health. 18.
Namwat, C., Suphanchaimat, R., Nittayasoot, N. and Iamsirithaworn, S. 2020. Thailand’s response against coronavirus disease 2019: challenges and lessons learned. Outbreak, Surveillance, Investigation & Response (OSIR) Journal. 13: 33-37.
Siamrath Online news. 2021. New 31 indigenous Covid-10 cases in Chiang Mai Province. Accessed 20 July 2021, from https://siamrath.co.th/n/260587.
Thaikruea, L. 2016. University engagement: A case study of Thailand’s first toxic jellyfish networks and knowledge establishment. Bangkok: Civil Society Engagement Thailand. 26-9.
Thaikruea, L. 2020. A Decade of Fighting Box Jellyfish Health Issues. p. 145-63. In M. Indrawan (ed) Civic Engagement in Asia: Lessons from transformative Learning in the Quest for a Sustainable Future.Yayasan Pustaka Obor, Jakarta.
Thaikruea, L. and Siriariyaporn, P. 2018. Injuries and deaths caused by toxic jellyfish: surveillance, prevention, and treatment, D-library national library of Thailand. Accessed 20 May, 2021, from from: http://164.115.27.97/digital/items/show/12987.
World Health Organization. 2020a. Global surveillance for COVID-19 caused by human infection with COVID-19 virus: interim guidance, 20 March 2020. Accessed 21 April 2021, from https://apps.who.int/iris/handle/10665/331506.
World Health Organization. 2020b. In-house real-time RT-PCR assays for the detection of SARS-CoV-2. Accessed 21 April 2021, from https://www.who.int/docs/default-source/coronaviruse/real-time-rt-pcr-assays-for-the-detection-of-sars-cov-2-institut-pasteur-paris.pdf?sfvrsn=3662fcb6_2.
World Health Organization.2020c. Laboratory testing strategy recommendations for COVID-19: interim guidance, 21 March 2020. Periodical Laboratory testing strategy recommendations for COVID-19: interim guidance, 21 March 2020, Accessed 21 April 2021, from https://apps.who.int/iris/handle/10665/331509.
World Health Organization. 2020d. WHO Director-General's opening remarks at the media briefing on COVID-19. Accessed 21 April 2021, from https://www.who.int/dg/
speeches/detail/who-director-general-s-opening-remarks-at-the-media-briefing-on-covid-19.
World Health Organization. 2021. Coronavirus disease (COVID-2019) situation reports. Accessed 21 April 2021, from https://www.who.int/emergencies/diseases/novel-coronavirus-2019/situation-reports.
Yano, T., Trakarnsirinonb, P., Kaewpintac, B., Chaisowwongd, W., Pattamakaewe, S. and Srikitjakarn, L. 2016. Performance of community volunteers in human and animal diseases surveillance by using mobile application. International Journal of Infectious Diseases 53: 121.
Yano, T., Phornwisetsirikun, S. Susumpow, P., Visrutaratna, S., Chanachai, K., Phetra, P. et al. 2018. A Participatory System for Preventing Pandemics of Animal Origins: Pilot Study of the Participatory One Health Disease Detection (PODD) System. JMIR Public Health Surveill. 4: e25.
Yu, F., Du, L., Ojcius, D. M., Pan, C. and Jiang, S. 2020. Measures for diagnosing and treating infections by a novel coronavirus responsible for a pneumonia outbreak originating in Wuhan, China. Microbes Infect. 22: 74-9.
OPEN access freely available online
Chiang Mai University Journal of Natural Sciences [ISSN 16851994]
Chiang Mai University, Thailand
https://cmuj.cmu.ac.th